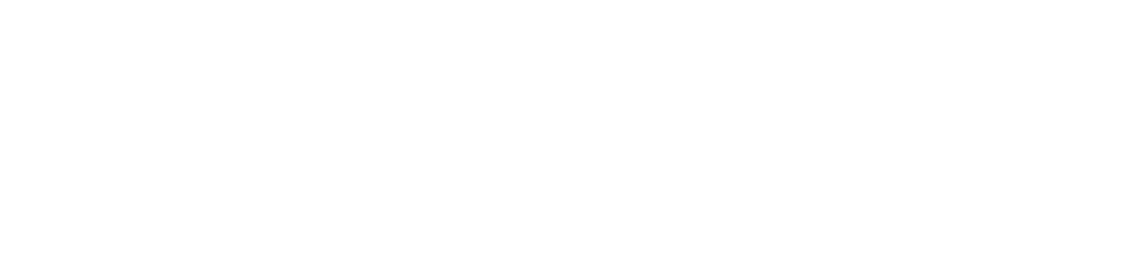
Intersectional Fairness (ISF) is a bias detection and mitigation technology for addressing intersectional bias, which is caused by the combinations of multiple protected attributes.
ISF leverages existing single-attribute bias mitigation methods to ensure fairness in machine-learning models when it comes to intersectional bias.
The approaches applicable to ISF include pre-, in-, and post-processing. Currently, ISF supports Adversarial Debiasing, Equalized Odds, Massaging, and Reject Option Classification.
Intersectional Fairness (ISF) is a sandbox-stage project of LF AI & Data Foundation.
Contributed by Fujitsu Limited in June 2023.